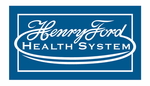
Title
Prostate and Prostate Cancer Segmentation Using a Deep Learning-Based Object Detection Algorithm
Files
Program
Radiation Oncology
Training Level
Resident PGY 3
Institution
Henry Ford Hospital
Abstract
Purpose: Mask-RCNN has been proposed in other industries for structure mapping and recognition. We attempted to use this platform for normal prostate segmentation and dominant intraprostatic lesion (DIL) segmentation and localization on multi-parametric MRI (Mp-MRI). This can potentially aid in diagnosis and therapeutic planning, as precise localization of the site of disease can guide targeted surgical resection or radiation boost volumes.
Methods: A total of 78 patients with biopsy proven prostate adenocarcinoma and MRI imaging were reviewed. Of these, 54 patients were used as a training set. The remaining 24 patients were selected for normal prostate segmentation. This cohort was then evenly divided into validation and testing sets. For the DIL localization and segmentation review, 57 patients were used as a training set. Of the remaining 21 patients, 11 were included in the validation set and 10 were used for the testing set. Mp-MRI images, including T2-weighted (T2W) and apparent diffusion coefficient (ADC) images were adopted for our experiment. T2W images alone were used in the normal prostate segmentation task and co-registered ADC maps to T2W images with the prostate patch cropped out were used in the DIL detection and segmentation task. All images were normalized, the histogram was equalized, and images were resized and padded slice by slice to a fixed size of 384 × 384 prior to the training, validation and testing phase. The normal prostate and DILs were contoured by a radiation oncologist from our institution. The DIL was separately contoured for 20 patients by a second radiation oncologist to assess for interobserver variability.
Results: Sensitivity and specificity for prostate segmentation were evaluated for all slices of patient imaging and was calculated to be 0.94 and 0.92, respectively. The dice similarity coefficient (DSC) was 0.87±0.04. The 95% hausdorff distance was 6.12 ±2.39 (mm). To evaluate the performance of Mask-RCNN, we used 2D and 3D Unet benchmarks, which had the DSC of 0.85±0.03 and 0.83±0.07, respectively. For the DIL segmentation, our overall detection rate was 81%, and for those detected lesions, the DSC was 0.64±0.14. The DSC of the contours between two clinicians was 0.67±0.21.
Conclusion: Our results demonstrate that the Mask-RCNN model can produce high quality segmentation results for prostate recognition on standard MRI images and can potentially aid in detecting and segmenting prostate cancer on Mp-MRI images. There was also similar interobserver variability on the DIL segmentation using Mask-RCNN. These results can potentially influence therapeutic options, such as targeted resection or intraprostatic boost volumes.
Presentation Date
5-2019
Recommended Citation
Feldman, Aharon; Dai, Zhenzhen; Carver, Eric; Liu, Chang; Lee, Joon K.; Pantelic, Milan; Elshaikh, Mohamed A.; and Wen, Ning, "Prostate and Prostate Cancer Segmentation Using a Deep Learning-Based Object Detection Algorithm" (2019). Clinical Research. 54.
https://scholarlycommons.henryford.com/merf2019clinres/54
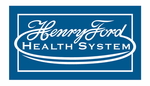