Files
Download Full Text (556 KB)
Program
Radiation Oncology
Training Level
Fellow
Institution
Henry Ford Hospital
Abstract
Purpose: Gleason Grading (GG) Grouping system is an important index in determining treatment plan or predicting outcome for prostate cancer patients. Unfortunately, currently GG Grouping results can only be obtained from biopsy-driven pathological tests. We aim to predict GG groups for PCa patients from multiparametric magnetic resonance images (mp-MRI). Methods: The challenges include data heterogeneity, small sample size and highly imbalanced distribution among different groups. A retrospective collection of 201 patients with 320 lesions from the SPIE-AAPM-NCI PROSTATEx Challenge (https://doi.org/10.7937/K9TCIA.2017.MURS5CL) was studied, among which only 98 patients with 110 lesions having GG available. And number of lesions from each group was 36, 39, 20, 8, and 7, respectively, for GG 1-5. We approached the challenging task by bridging though easier one of classifying 320 lesions into benign or malignant, and transferring learned knowledge to GG prediction on 110 lesions. During implementation, a four-convolutional neural network (CNN) was used for malignancy classification. To prevent over-fitting on small sample size, instead of fine-tuning on CNN, learned features were extracted and classified by weighted extreme learning machine (wELM), traditional classifier that assigned larger weight to samples from minority class.Image pre-processing included registration and normalization. Image rotation and scaling were also used to increase sample size and re-balance number of malignant and benign lesions. Results: The best combination of modalities as input to CNN was found to be T2W, apparent diffusion coefficient (ADC) and B-value maps (b=50 s/mm2). During phase 1 of CNN training, average and best results of (Sensitivity, Specificity, G-mean) over 10 folds were (0.53, 0.83, 0.65) and (1, 0.88, 0.91), respectively. Features from best performing model were extracted to represent each lesion, and those from the last convolutional layer were found constantly better than from all other layers (Table 1). This implies that semantic features regarding lesion information is more important than local and detailed features such as contrast change in GG prediction. Conclusion: This work has successfully tackled the challenging task of GG prediction from mp-MRI by bridging through an easier task and has combined feature extraction using deep learning model and small data classification using traditional classifier to benefit from both.
Presentation Date
5-2019
Recommended Citation
Lee, Joon K.; Zong, WeiWei; Pantelic, Milan; and Wen, Ning, "Gleason Grade Group Prediction for Prostate Cancer Patients with MR Images Using Convolutional Neural Network" (2019). Basic Science Research. 5.
https://scholarlycommons.henryford.com/merf2019basicsci/5
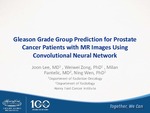